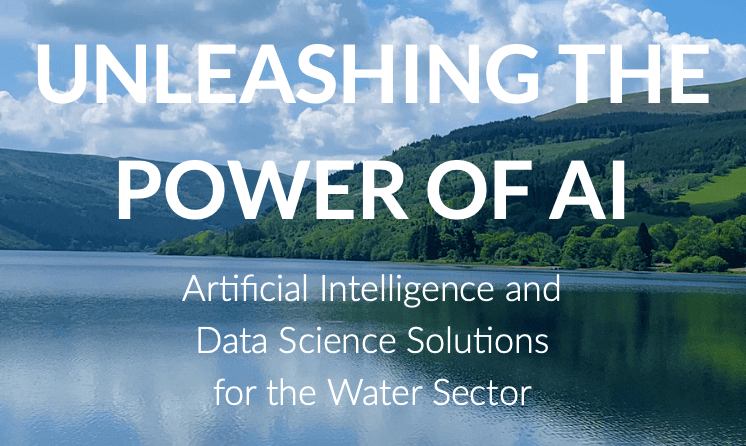
- INTELLIGENCE
- …
- INTELLIGENCE
- INTELLIGENCE
- …
- INTELLIGENCE
WORKING WITH US
What a typical project process will look like
Option 1 – Solution exploration and initial data assessment
If you have an idea or a data science challenge that you believe may solve a problem or improve a process we will work with you to scope a new data application and assess the feasibility of the idea including a high-level review of your data and high-level cost benefit appraisal.
Typically no charge, no commitment.
Option 2 – Create Proof of concept for a new solution.
We produce a functioning prototype solution to demonstrate the feel, functionality, and potential value of your data solution. Use this to prove the application in your organisation and decide whether a wider roll-out is suitable.
Typically a fixed price engagement depending on complexity/scope of the solution.
Option 3 - Full solution adoption
Usually following a Proof of Concept. We provide a fixed price quote for the creation and implementation of your solution . In addition to traditional purchase (fixed price + licence), we can also offer a complete SaaS option (monthly fee, low commitment) for most new solutions.
Our existing solutions can also be adapted and configured to suit your circumstances. SaaS and traditional price options available.
To find out more about how Collaborate Water can use the power of your data to transform your operations:
Email us: info@collaboratewater.com
Sign up for our newsletter:
© Copyright-2023 Collaborate Water - Privacy Policy